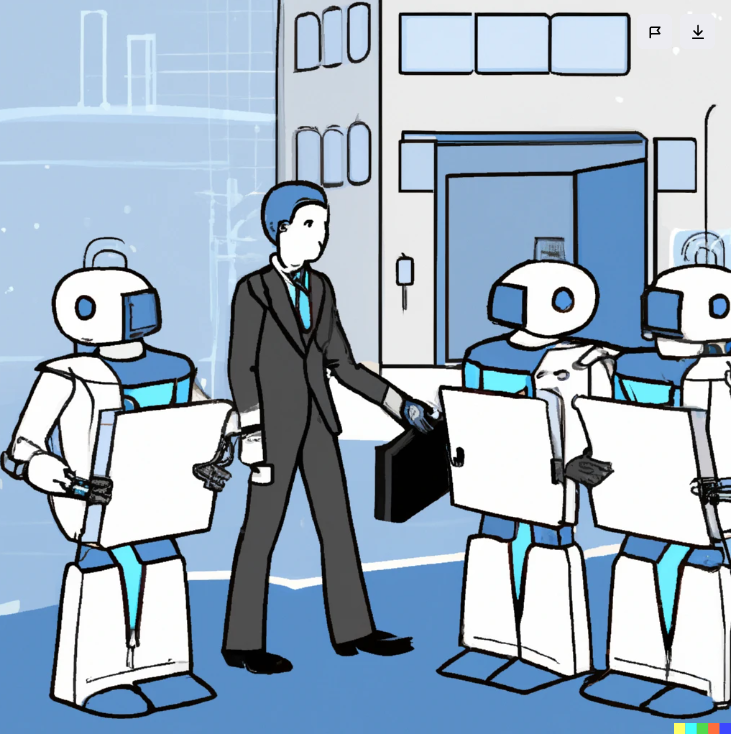
When does AI pay off? AI-adoption intensity, complementary investments, and R&D strategy – a study of high-tech ventures in South Korea. The study finds that AI is associated with higher revenue growth only at higher levels of adoption. Since AI is ultimately dependent on data, this growth is much more evident especially when companies leverage complementary technologies such as cloud computing.
The digitalisation paradox of everyday scientific labour: How mundane knowledge work is amplified and diversified in the biosciences – fascinating ethnographic research on a synthetic biology research group. The authors argue that:
Contrary to expectations of the removal of mundane work by automation and digitalisation, we suggest the emergence of a digitalisation paradox in knowledge-intensive, creative professions such as scientific work. We argue that while robotics and advanced data analytics in scientific work aim at simplifying work processes so as to increase productivity, they can also contribute to increasing the complexity, number, and diversity of tasks, and that this happens unevenly across the scientific hierarchy.
Artificial intelligence in science: An emerging general method of invention – a study of the adoption of deep learning in the sciences. Particularly, they explored the impact of neural networks in the health sciences through both the citations of the studies and also recombinational novelty (measured through the distance of cited journals). In terms of citation impact, they find high variation across studies adopting deep learning. In terms of novelty, they find a negative association. The authors then suggest that deep learning has been used primarily to manage the increasing amount of data within a field, instead of using it to synthesize knowledge across domains.
Artificial intelligence as an enabler for innovation: A review and future research agenda – a special issue from Technological Forecasting and Social Change exploring how AI can enable innovation across idea generation, screening, experimentation, development and commercialization.
Machines augmenting entrepreneurs: Opportunities (and threats) at the Nexus of artificial intelligence and entrepreneurship – explores how entrepreneurship researchers can engage with the advances in AI. They highlight the following changes that entrepreneurs need to be ready to capitalize:
- As society transitions toward a “feeling economy,” entrepreneurs can leverage AI to aid in recognizing, communicating and responding to emotions
- With AI transforming jobs and creating new jobs, entrepreneurs need to adapt as occupational skills get redistributed across the economy.
- Entrepreneurs would need to be active in developing new governance mechanisms to ensure that AI does not harm society.
- Entrepreneurs would have to conceptualize what would be the role of humans in the decision process as AI becomes more prevalent.
- Entrepreneurs have to expand the role of humans in developing AI systems.
- As a tool, AI should be directed by society towards the good.